Monitoring Azure Service Bus (SB) comes with its own set of challenges, primarily due to the distributed nature of the service and the complexities involved in message processing and delivery. Some of the most common challenges associated with monitoring Azure SB include:
Message Flow Monitoring: Tracking the flow of messages through various queues or topics, including understanding where bottlenecks might occur or where messages might be delayed.
Latency Monitoring: Ensuring that messages are being processed within acceptable time frames.
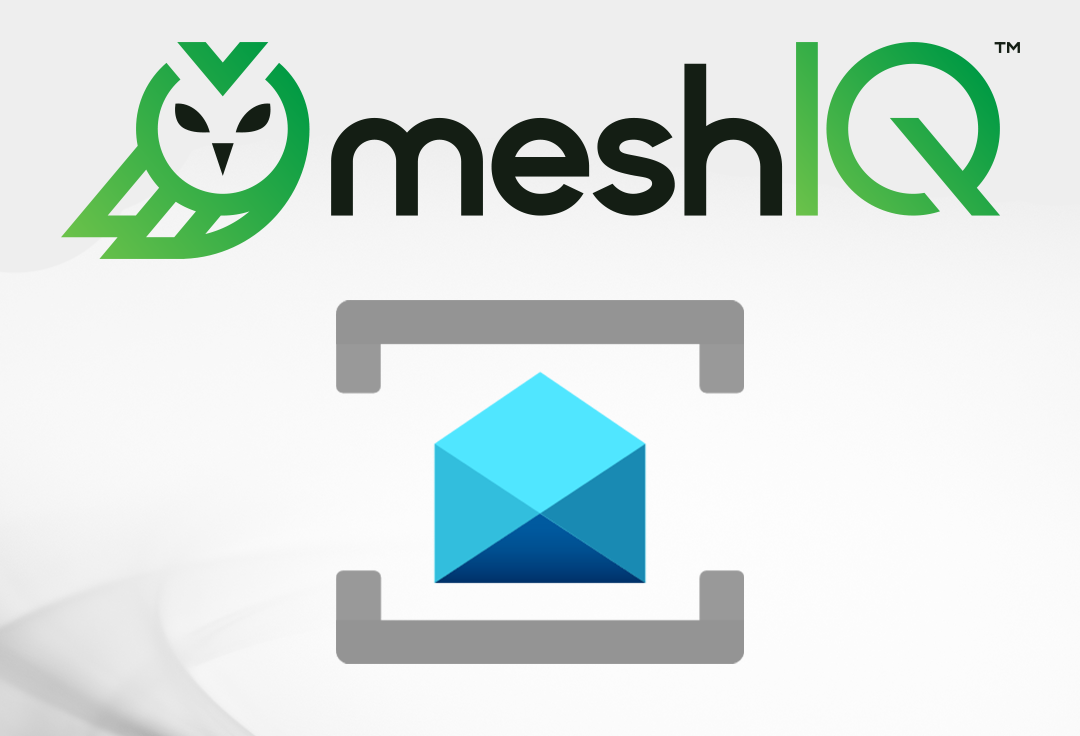